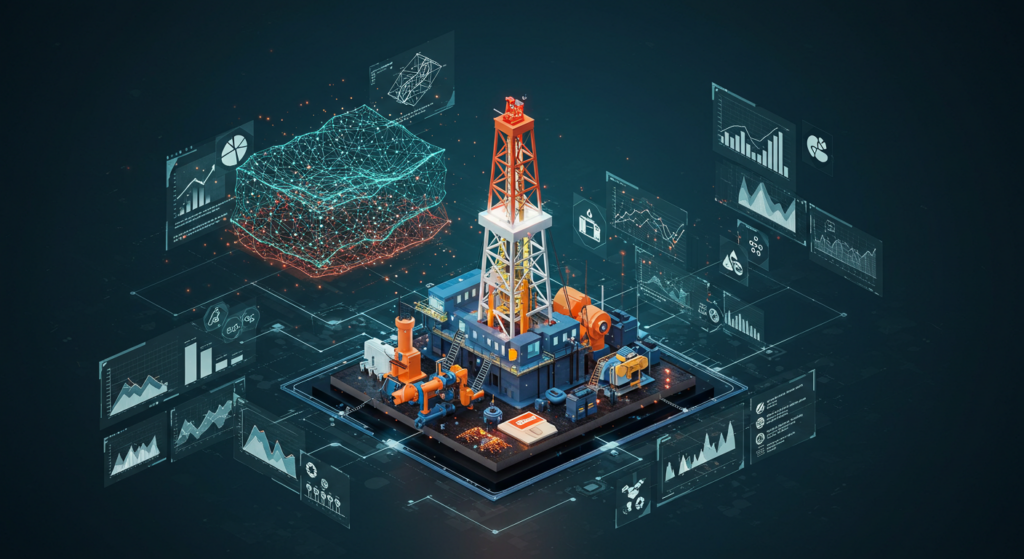
The oil and gas industry, long characterized by its reliance on manual processes and legacy systems, is undergoing a digital revolution. Artificial Intelligence (AI) and data analytics are driving unprecedented efficiencies, cost savings, and sustainability improvements across the sector. From predictive maintenance to reservoir optimization, these technologies are reshaping how companies explore, extract, and deliver energy resources. In this article, we explore the key applications of AI in oil and gas, the impact of data analytics on operational efficiency, and emerging trends that are defining the future of this critical industry.
1. Predictive Maintenance: Minimizing Downtime and Maximizing ROI
One of the most impactful applications of AI in the oil and gas sector is predictive maintenance. Equipment failures in drilling rigs, pipelines, or refineries can lead to costly downtime, safety risks, and environmental hazards. By leveraging machine learning algorithms and IoT sensors, companies can analyze real-time data to predict equipment malfunctions before they occur. For example, AI models trained on historical maintenance data can identify patterns indicative of wear and tear. Sensors embedded in machinery monitor variables like temperature, vibration, and pressure, flagging anomalies that signal potential breakdowns. This proactive approach reduces unplanned outages by up to 30%, according to industry reports, while extending asset lifespans.
2. Reservoir Management and Exploration Optimization
Locating viable hydrocarbon reserves is a high-risk, high-cost endeavor. Traditional methods rely heavily on seismic surveys and geological expertise, but AI-powered reservoir simulation is revolutionizing exploration. Advanced algorithms process vast datasets—including seismic data, well logs, and production history—to generate precise 3D models of subsurface reservoirs. Companies like Shell and BP are using machine learning for reservoir characterization, enabling faster decision-making and reducing exploration costs by up to 20%. AI also enhances recovery rates by identifying optimal drilling locations and predicting reservoir behavior under different extraction scenarios.
3. Drilling Optimization: Precision and Cost Efficiency
Drilling operations account for nearly 50% of upstream costs, making efficiency a top priority. AI-driven drilling optimization tools analyze real-time data from rigs to adjust parameters like weight-on-bit, rotational speed, and mud flow. This ensures smoother drilling, minimizes tool wear, and prevents costly non-productive time (NPT). Autonomous drilling systems, powered by AI and robotics, are also gaining traction. These systems reduce human error and enable continuous operations in harsh environments. For instance, Equinor’s autonomous drilling platform in the North Sea reported a 15% reduction in drilling time using AI-guided systems.
4. Enhancing Safety and Environmental Sustainability
Safety and environmental stewardship are critical concerns for the oil and gas industry. AI and data analytics play a pivotal role in mitigating risks. Computer vision systems monitor worksites for safety compliance, detecting hazards like gas leaks or unauthorized personnel in restricted zones. Moreover, AI helps reduce the industry’s carbon footprint. Predictive analytics optimize flaring and venting processes, while machine learning models improve energy efficiency in refineries. Companies are also using AI for methane emissions detection, partnering with satellites and drones to identify leaks faster than traditional methods.
5. Supply Chain and Demand Forecasting
Volatile oil prices and shifting market demands necessitate agile supply chain management. Data analytics in oil and gas enables accurate demand forecasting by analyzing historical trends, geopolitical events, and economic indicators. AI models predict price fluctuations, helping companies optimize inventory levels and refine production schedules. During the COVID-19 pandemic, firms like Chevron used AI to recalibrate supply chains in response to collapsing demand, avoiding billions in losses. Today, AI-driven logistics optimization ensures timely delivery of equipment and materials, even in remote locations.
6. Digital Twins: Virtual Replicas for Real-World Insights
The concept of digital twins—virtual replicas of physical assets—is gaining momentum. By integrating AI, IoT, and real-time data, companies create dynamic models of pipelines, refineries, or entire offshore platforms. These digital twins simulate scenarios like equipment failures or process changes, enabling engineers to test solutions without disrupting operations. ExxonMobil, for example, uses digital twins to monitor its global fleet of LNG carriers, optimizing fuel consumption and reducing emissions. This technology is a cornerstone of the industry’s digital transformation, bridging the gap between physical and digital operations.
Emerging Trends and Future Outlook
As the industry embraces Industry 4.0, several trends are shaping its trajectory:
- Edge Computing: Processing data at the source (e.g., on drilling rigs) reduces latency and enhances decision-making speed.
- Blockchain for Transparency: Secure, decentralized ledgers improve traceability in supply chains and carbon credit trading.
- AI Ethics and Governance: With growing reliance on AI, frameworks for ethical data use and algorithmic accountability are becoming essential.
Embracing the AI-Driven Energy Future
The integration of AI and data analytics in the oil and gas industry is no longer optional—it’s a strategic imperative. Companies that adopt these technologies gain a competitive edge through cost savings, operational resilience, and alignment with global sustainability goals. From predictive maintenance to carbon reduction, AI is unlocking value across the entire value chain. As the sector navigates challenges like energy transition and workforce digitization, investing in machine learning, IoT, and big data analytics will be key to thriving in an era of unprecedented change.
Learn more about Data Analytics, Machine Learning & Artificial Intelligence with EnergyEdge.
Find upcoming courses below:
Budgeting & Forecasting In The Artificial Intelligence Era – Empowering Energy Finance Professionals
Digital Twin Engineering – From Concept to Deployment in Oil & Gas Operations